Fuel efficiency and sustainability are critical concerns in the aviation industry, requiring innovative approaches to address them. With the increasing demand for air travel, managing this growth efficiently becomes crucial to minimizing the environmental footprint. Optimizing flight paths, especially through the implementation of Continuous Descent Operations (CDO), offers a significant opportunity to reduce fuel consumption and carbon emissions. By leveraging artificial intelligence (AI), we can further enhance these outcomes predicting optimal routes to manage air traffic more effectively. This approach is both a step towards more sustainable aviation and a necessary evolution in the face of increasing complexity in air traffic management.
Strengthening Air Traffic Management through collaboration,
The collaboration between Air Traffic Management Research Institute (ATMRI) and AIR Lab signifies a vital link between theoretical research and its practical application in the field AI and air traffic management (ATM).
Established by the Civil Aviation Authority of Singapore (CAAS) and Nanyang Technological University (NTU) in 2013, ATMRI aims to innovate and transform air traffic management in the region, aligning with Singapore’s vision as a global center of excellence. Research domains encompass Deep Digital Towers, Controller-AI teaming, multi-modal data analytics, urban aerial transport, and emerging technologies like Human-AI hybrid systems, large language models and conformal automation.
AIR Lab, a joint lab established by CAAS and Thales in 2019, focusing on the development of an Open ATM system architecture. AIR Lab has been exploring advanced and open technologies to advance the future of air traffic management. AIR Lab has been exploring advanced and open technologies to advance the future of air traffic management through the development of a digital data platform to support research and the prototyping of green aviation proof of concept such as CDO (Continuous Descent Operation), by leveraging Open Architecture and Artificial Intelligence. The AIR Lab brings together multiple stakeholders in the ATM value chain – from air traffic controllers to AI experts, to software engineers and local start-ups.
The AIR Lab’s ATM expertise, together with ATMRI’s research on AI and ML, spearheads the use of open and advanced technologies to improve air traffic management systems.
AI & Machine Learning’s roles enhancing the Continuous Descent Operation & Top Of Descent,
As defined by ICAO, ‘’CDO is an aircraft operating technique aided by airspace design, procedure design and appropriate ATC clearances, enabling the execution of a flight profile optimized to the operation capability of the aircraft, with low engine thrust settings and where possible, a low drag configuration, thereby reducing fuel burn and emissions during descent. ‘’
With this approach, the aircraft remains higher for longer and operates at lower engine thrust. CDO can lead to the reduction in noise, atmospheric emissions, fuel-use and ease pilot and controller communication.
To calculate a perfect Top of Descent (TOD) for a Continuous Descent Operation (CDO), it’s essential to integrate various factors – such as the aircraft type, weather, TMA constraints, traffic patterns airspace entry point… The objectives are to predict the optimal TOD and contribute to establishing a fuel-efficient CDO profile.
The application of machine learning (ML) enables learning from various sources, such as successful Continuous Descent Operations (CDO), human-level performance, historical data, and previous decisions. By integrating with Air Traffic Management System (ATMS) data, including Terminal Maneuvering Area (TMA) traffic characteristics, ML not only leverages existing knowledge but also continually enhances and refines its capabilities. This improvement is also driven by analyzing outcomes like unsuccessful CDOs, AI agent performance, and decisions from new trials, facilitating a deeper understanding and more effective decision-making processes.
Researchers from ATMRI focus on the potential of leveraging artificial intelligence (AI) and machine learning (ML) in refining the calculations of the TOD for CDO, for a potential integration of the prediction model with Open ATMS.“Applying AI and ML technologies can significantly improve TOD calculations for CDO, leading to a more precise and fuel-efficient approach in air traffic management“ ATMRI researchers. This focus on AI and ML underscores a broader shift in the aviation industry towards smarter, greener, and more efficient operations.
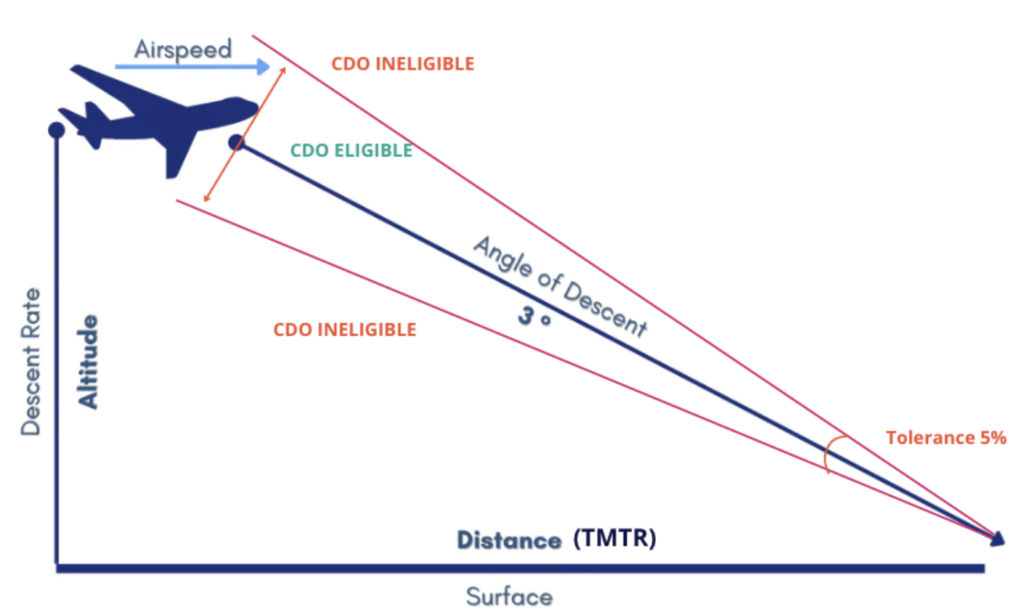
Value outcomes achieved from the collaboration between ATMRI and AIR Lab
The collaboration between AIR Lab and ATMRI enables the translation of complex academic findings into actionable, real-world implementations, highlighting the importance of integrating research insights with operational practices. This partnership not only accelerates the advancement of air traffic management technologies but also underscores the value of combining academic research with industry expertise to address the challenges of modern air traffic systems. Through this collaboration, AIR Lab and ATMRI demonstrate how innovative research can lead to significant improvements in the efficiency and safety of air navigation services, establishing a new benchmark for excellence in the sector.